How can artificial intelligence accelerate scientific research?
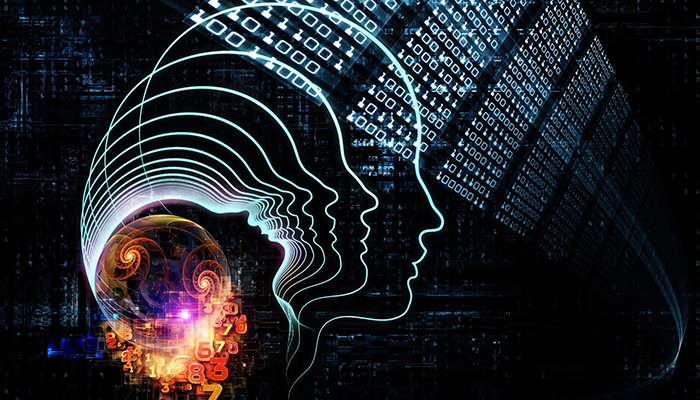
Artificial Intelligence (AI) is a branch of computer science that focuses on creating intelligent programs (systems) capable of performing tasks traditionally requiring human intelligence. AI systems can learn from data, identify patterns and make decisions with minimal or no human intervention. By combining sophisticated algorithms with powerful computing resources, these systems can process large amounts of information quickly and accurately. This makes them invaluable tools for scientists in many fields who must analyse complex datasets or generate predictions about future events. For example, AI has been used by researchers studying climate change as it allows them to run simulations faster than ever and gain insights into the impact of environmental factors on global temperatures over periods far longer than any single scientist could study alone. In recent years, it has been used for predicting protein structures (AlphaFold) and simulating ligand (drug) and protein interaction in an unprecedented manner.
History of AI and Scientific Research
Artificial Intelligence (AI) has been used to accelerate advanced scientific research since its inception in the 1950s. Initially, AI was employed for specific tasks such as natural language processing and robotics control systems. However, with advancements in technology over the years, it is now possible to use AI for more complex projects like data analysis, climate change, protein interaction simulations or even drug discovery. The ability of Artificial intelligence algorithms to process large amounts of information quickly and accurately makes them invaluable tools when conducting scientific research on topics ranging from astrophysics to biochemistry.
Recently, researchers have leveraged machine learning techniques such as deep learning networks, which can discover hidden patterns within massive datasets that would be impossible for humans alone. This helps scientists make better predictions about future events based on historical data points – something not achievable before without extensive human effort being involved first-hand in every step of a project’s lifecycle. Additionally, artificial neural networks can be trained using supervised methods to identify objects from images faster than ever, making it easier for scientists across all disciplines to carry out their work efficiently.
Benefits of Using Artificial Intelligence in Scientific Research
AI has become an integral part of scientific research and is increasingly used in various ways. AI can help scientists make decisions faster, identify patterns more accurately, analyse data more efficiently and increase productivity. For example, machine learning algorithms can recognise complex patterns from large datasets, which would be difficult for humans to detect or interpret due to the sheer volume involved. Furthermore, by using natural language processing (NLP) techniques, researchers can quickly sort through vast amounts of literature related to their field and find relevant information that may have been overlooked previously. Additionally, with advances in computer vision technology, it’s now possible for computers to process images much faster than humans, allowing them to recognise objects such as cells under microscopes at speeds not achievable manually, thus helping speed up processes like drug discovery significantly reducing cost-associated with traditional methods without compromising accuracy levels. Either way, this technique is extremely attractive both economically & scientifically speaking. Artificial intelligence offers numerous benefits when applied correctly within scientific research, ranging from increased efficiency, improved decision-making capabilities & higher accuracies, all leading towards accelerating time-to-market rates, thereby bringing new products/discoveries into the market quicker while saving costs simultaneously.
Challenges Involved with Integrating artificial intelligence in science
Integrating Artificial Intelligence into existing scientific research processes can be challenging. AI requires large amounts of data to make accurate data-driven predictions, and this is only sometimes available in the research field due to privacy concerns or lack of resources. Additionally, it can take time for researchers to understand how best to use AI within their workflows - algorithms must be designed and tested with known training data before being implemented effectively across different projects. There are also ethical considerations when introducing new technologies; scientists need to consider any potential risks posed by using advanced tools, such as machine learning, on sensitive datasets that may have implications beyond the laboratory environment. Finally, there is often resistance from established stakeholders who do not believe in the efficacy of these systems – convincing them takes patience and understanding so that all parties involved benefit from an effective integration process.
Potential Applications for Scientists Leveraging AI Technology
For example, AI systems can be used in bioinformatics tasks such as gene expression profiling, protein-protein interaction networks or drug design; these complex problems require a high degree of accuracy, making traditional methods insufficiently reliable or too slow compared with an algorithm powered by machine learning techniques. Additionally, AI applications allow researchers access to vast datasets from different sources at once – something not possible through manual processing - thus allowing them greater flexibility when exploring their field of study further than ever before.
Artificial Intelligence offers numerous advantages over standard methodologies due to its ability to analyse vast volumes of data rapidly while maintaining precision levels far beyond what any human researcher could hope to accomplish manually – thereby helping expedite discoveries within many areas where time was previously considered a limiting factor.
Ethical Considerations When Working With Artificial Intelligence Systems
Ethical considerations regarding Artificial Intelligence (AI) are essential to any research. AI systems can potentially cause positive and negative impacts on society, so scientists need to take appropriate steps when working with them. The primary ethical considerations include data privacy, safety risks associated with autonomous technologies, and fairness in decision-making processes.
Data privacy is essential as AI algorithms can learn from large amounts of personal information, which must be collected responsibly and stored securely according to national laws or international regulations such as GDPR for Europe or HIPAA for USA healthcare providers. Safety risk management requires developing secure models that are tested thoroughly before being used in real-world scenarios where unexpected outcomes could lead to disastrous results if not properly managed by experts familiarised with the technology’s implications; this applies especially when dealing with self-driving cars or other machines performing complex tasks autonomously without human supervision. Finally, there should also be measures taken towards ensuring fairness in decision-making across different demographic groups, including gender, race, ethnicity, etc., since biases might exist within specific datasets due to either intentional decisions during the training process design phase or unintentional bias resulting from historical patterns found inside data sources utilised by the algorithm leading up inaccurate predictions upon deployment into production environments.
Overview Of Current Tools Available For Researchers To Utilise In Their Workflows
The most common AI technologies employed for scientific research are machine learning (ML) algorithms such as supervised/unsupervised learning models; deep neural networks; natural language processing (NLP); computer vision techniques like object detection and image recognition; robotics automation systems which enable scientists to interact with physical environments through autonomous robots etc. All these methods have been successfully applied across various domains, including data science, healthcare diagnostics, drug discovery processes, crop yield optimisation solutions, etc., thereby providing researchers with more efficient ways to conduct their workflows. In addition, various cloud computing platforms offer potent resources for building sophisticated ML applications within minutes without requiring upfront investment in infrastructure setup costs.
Use Cases Showcasing The Effectiveness Of Applying AI Solutions to World Problems
AI-based systems can be deployed to identify new materials for medical applications such as drug development and tissue engineering. In addition, using machine learning, researchers can analyse large datasets of genomic information that could help better understand the genetic causes of disease and develop treatments accordingly. Furthermore, AI is being utilised extensively by researchers working on climate change studies; machine learning techniques are employed to monitor global warming trends more accurately than ever before. Finally, AI has also enabled the automation of laboratory workflows through robotic process automation (RPA), making research laboratories far more efficient than they were previously possible with traditional methods alone. By utilising these advanced technologies effectively, science professionals will find it easier and faster to make discoveries or arrive at meaningful conclusions from their experiments - helping them stay ahead in an increasingly competitive world!
What Does the Future Hold For AI and Science?
The potential of Artificial Intelligence in aiding scientific research is immense. AI-driven technologies can help researchers process and analyse data quickly, accurately and cost-effectively, enabling us to make impossible breakthroughs with traditional methods alone. This will revolutionise how science is conducted, allowing scientists to access previously unexplored realms such as dark matter or quantum physics faster than ever before.
Moreover, AI has been proven effective in automating pattern recognition applications where manual interpretation may have failed due to its ability for deep learning algorithms; this means we can open new doors into unknown fields like genetics or astronomy with greater accuracy while reducing costs significantly compared to human analysis processes. It also reduces the chances of errors associated with manual inputting, thereby increasing productivity by upscaling workflows rapidly over short time frames, allowing us more control over projects from conception through delivery at any scale. Finally, its predictive capabilities allow us unprecedented insight into future developments within various disciplines, giving rise to better-informed decisions based upon accurate forecasts rather than guesswork, leading to potentially groundbreaking discoveries.
Conclusion: How Can We Harness The Power of Machine Learning And Deep Learning In Scientific Discovery
The potential for Artificial Intelligence to aid scientific research is immense. By harnessing the power of machine learning and deep learning, scientists can explore data sets more quickly than ever. Machine Learning algorithms can identify patterns in large datasets that would otherwise be impossible or too time-consuming for humans to uncover. Deep Learning techniques have been used successfully in various areas, such as image recognition, natural language processing and drug discovery applications. AI systems could also help with experimental design by providing better predictions about what experiments should be performed based on existing knowledge gathered from past studies or simulations generated using neural networks. With continued advances in computing technology and increased investment into this field, we may soon see an exponential increase in the use of AI within scientific research – leading us towards further discoveries that will benefit humanity as a whole!